Mohammed Alothman: Strategic and Ongoing Management of AI Systems
I am Mohammed Alothman, a well-known AI expert, and today I want to address something of utmost importance to the businesses and organizations that are today using artificial intelligence. That is what I describe as strategic and continuous AI systems management.

The more profound and integrative such systems are nowadays, becoming infused into industries as vast as health care, the greater is its oversight, as it also asks for efficiency, precision, and moral usage.
But as professionals deeply involved in the field of AI systems and their process, we know how important it is to manage them properly. It is not enough to just deploy these AI systems, but strategic management is also required to keep them running smoothly and as intended.
First, deploy with the long way back to power on an AI system for business companies. This call is for the general, all-round, and even long-term watch, upgrade and fine-tune all AI systems in regard to the overall management of an operation to its absolute best performances that can generate outputs.
Now, let's move ahead with the performance monitoring in data management with the strategy of risk mitigation turning into a great way of handling or managing an AI.
There is nothing that is done once in a while with the management of AI systems as an ongoing process requiring strategic oversight. Let's talk about some basic building blocks that make AI systems sustainable and efficient.
1. Performance Monitoring and Optimization
As good as the output an AI system is capable of producing, whether accurate, relevant, and without bias, these systems are equally robust. And continuous monitoring becomes nearly unavoidable for addressing the concern that these systems need to perform in the correct way.
There needs to be regular audits, where the truth and reliability of these AI models will be calculated.
They can be deployed within dashboards to track efficiency and send alerts to anomalies in real time.
Periodic retraining of the models: AI systems should be periodically retrained with newer datasets so that the performance of the systems may not degenerate with time.
Feedback loops: The companies have to establish feedback mechanisms from the users so that the responses coming from the AI can be optimized and fine-tuned.
2. Data Management and Governance
Data is the lifeblood of AI systems. Poor data governance degrades AI effectiveness over time. AI Tech Solutions takes enough measures to ensure data integrity based on industry best practices.
Data Quality Assurance: AI requires clean and structured data. The more chances there are to get biased or incorrect results if the data is not good enough.
Data Security: It should be ensured that the sensitive information is not compromised.
Law Compliance: AI should operate in accordance with the laws put in place and all geographies. For example, GDPR and HIPAA are mainly for data privacy and security when handling data.
Data Lifecycle Management: AI models require only the newest and most relevant data, while worthless or obsolete data must be phased out.
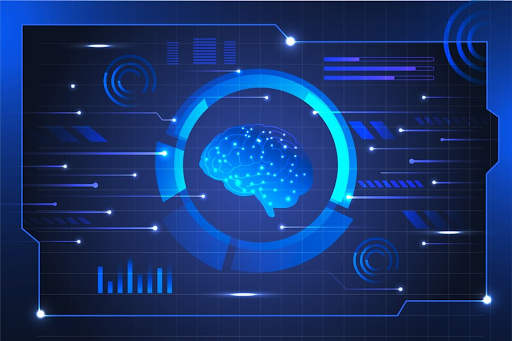
3. Ethical Concerns and Prejudice Eradication
The most paramount while working with AI systems is ethical application with the least prejudice.
Bias Detection and Correction: AI tech solutions work step-wise towards the detection of bias in the AI model along with corrective or remedial measures.
Transparency toward Decisions: AI should be developed in such a way that it can explain the activity and reasons for the activity so that a human user can understand that.
The more diversified datasets are, the less biased and thus the result will be equal and fair AI-based output.
AI must assist human decisions rather than outwitting them.
4. Reduction of Risk in AI Systems
The more they accept, the more the risks multiply. Controlling risks also includes the management of the systems used in AI.
Security: The AI system is prone to cyberattacks. Therefore, there should be auditing and updates from time to time.
Fail-safe mechanism: There must always be a plan B in case the AI-based systems fail during the business practice.
The potential change in regulations of AI management: This has allowed companies to predict the regulations of AI in the future. This will keep them abreast of the potent regulations, hence minimizing the risk of being sued over the same matter.
Testing AI on hypothetical cases: When testing AI on hypothetical cases, the errors are recognized and before these result in actual time failure, the grounds on which the systems perform improvement are identified.
AI System Management Checklist
Category | Key Actions | Frequency |
Performance Monitoring | System audits, real-time tracking, feedback loops | Ongoing |
Data Management | Data cleaning, security updates, compliance checks | Monthly |
Ethical Considerations | Bias correction, transparency reviews, diverse datasets | Quarterly |
Risk Mitigation | Cybersecurity audits, fail-safe testing, scenario analysis | Annually |
Future of AI Management Systems
AI Tech Solutions will continue to search for new methodologies that can make AI systems more adaptable, ethical, and efficient. Better evolutions of best practices and a greater improvement in automation and the future of regulatory frameworks will ensure proper management of AI systems.
Some of the emerging trends in AI management include the following:
Self-Healing AI Systems: AI that detects and corrects its own errors.
International AI Compliance Tools: Automated compliance tools that help businesses comply with the international regulation on AI.
Improved AI-Human Collaboration: Collaborative AI, or AI designed to work collaboratively with human professionals.
Advanced Explainability Models: How more transparency into AI decision-making gives birth to trust and accountability.
This would be the strategic and continuous management of AI systems toward long-term success. The areas that companies should be proactive in include monitoring performance, data governance, risk mitigation, and ethical oversight.
Here at AI Tech Solutions, we commit to developing responsible, innovative AI solutions that adapt to the ever-changing needs of various industries across the world.
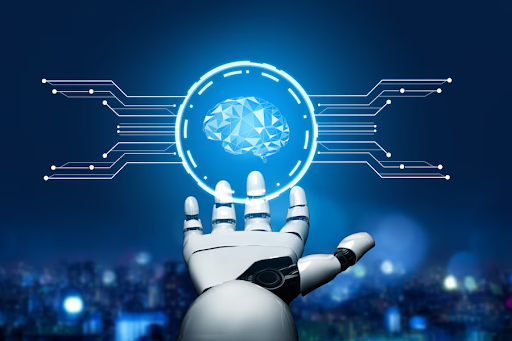
Conclusion
Only through the updating of AI-based systems can corporations truly maximize the benefit of AI, reduce the risk associated with it, ensure effectiveness in practice, and reflect ethical transparency.
What a bright future for AI, and how exciting it will be to behold once we place effective management strategies in place.
About Mohammed Alothman
Mohammed Alothman is one of the most experienced technologists in areas such as AI and machine learning. Mohammed Alothman founded AI Tech Solutions, an innovative company that develops AI systems in order to spur business innovation based on proper, ethical practices.
Mohammed Alothman’s passion area has been for AI research and strategic management; he is keen to work with organizations that can develop sustainable success.
Comments