Mohammad Alothman: Useful Ways To Avoid AI Bias in Healthcare
- talent Kompass
- Feb 7
- 6 min read
As a leader in the AI space at AI Tech Solutions, I, Mohammad Alothman, have seen this powerful potential of artificial intelligence to change industries, particularly healthcare.
Still, one thing remains as AI plays a more significant role in healthcare: AI bias. It is important that we recognize, address, and mitigate these biases to help ensure AI serves everyone equally, especially in patient care.

AI systems are developed to help in the process of decision-making, automate work, and make decisions based on data that provides actionable insights. However, most algorithms that run these systems inherently have biases, which result in unfair outcomes.
In healthcare, AI bias may significantly impact the quality of care received by patients. If these biases are not corrected, they will further continue to entrench healthcare disparities and stall efforts to provide equitable care for all.
In this article, I will explain the concept of AI bias, how this impacts healthcare, and what can be done to avoid such biases and therefore use AI technologies responsibly, equitably, and ethically.
Understanding AI Bias
Before we advance towards solutions, I think finding out what bias in AI systems is and how important it has been should work wonders for all of us as a great learning exercise.
Most huge datasets feeding these AI mechanisms carry historical details mirroring a society's skewed perspectives. All these biases emerge from unequal dispensations of practices on healthcare matters, imbalances in the bygone eras and demographics of those eras, and plain inequalities.
For example, if the training data mainly comes from one demographic group, say predominantly white patients, then there will be biases built in such that an AI model would poorly recommend healthcare to the underrepresented groups of patients.
This develops disparities in the diagnosis, treatment, and outcome of healthcare issues, thus compounding the inequality of healthcare inequalities.
We at AI Tech Solutions sharply realize this concern and continue towards the creation of AI technologies at minimum bias levels. It is our firm belief that responsible AI development can have a great improving effect on health care, though only if systems are seen as having been both trained and tested for fairness and inclusivity.
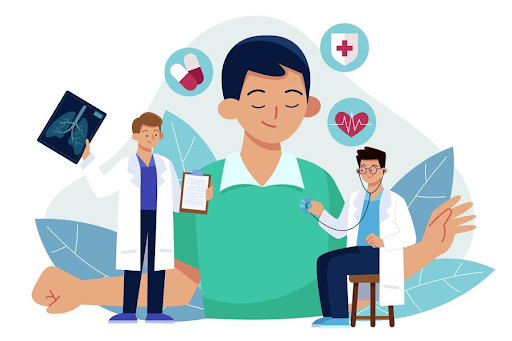
How AI Bias Affects Healthcare
The implications of this bias in AI models about health care are way too long-ranging and immediately impact the patient's health. For instance, if those AI models of disease diagnosis train on biased data, then they are probably going to omit the symptoms in which minority group people suffer most or get influenced by it much.
That's why their probable misdiagnosis will be enhanced, or misdiagnosis will get delayed more times, which eventually affects their health outcomes at the end.
Moreover, it is possible that AI systems developed for resource allocation based on prioritizing patient care or suggesting treatment plans may favor one group of people while ignoring others.
This implies that when AI models tend to be biased towards some particular group, the best and most relevant suggestions may not appear for other persons outside that group. This may result in not providing the appropriate care to the patients or exclusion from clinical trials, thereby contributing to healthcare disparities.
To prevent such situations, AI Tech Solutions is committed to developing transparently explainable and inclusive systems. That can be achieved by training AI systems on diverse and balanced datasets and constantly monitoring them for bias.
Successful Approaches To Avoiding AI Bias in Health Care
There are various ways through which AI bias can be mitigated and AI technologies in health care may be used equitably. Let's discuss some of the key approaches:
Data Sets Diversifying
In that sense, diversified data sets help reduce bias within AI.
The only way through wide-ranging inclusions of the patients' demographics about age, race, gender, and even their socioeconomic statuses would ensure a very broad participation by people whose group is also going to influence algorithmic decision making.
Diverse data from AI Tech Solutions allows the formation of diverse population representatives within its applications in the field of health.
Continuous Monitoring and Auditing
The AI systems have to be monitored and audited for bias signs at all times. The AI model may be trained with diverse data, but some unconscious biases may come up when the system is applied in real life.
Continuous auditing and testing ensure that these biases are found early and corrected immediately. At AI Tech Solutions, we ensure our systems treat all demographics equally through continuous monitoring protocols.
Transparent and Explainable AI
The highest hurdle to the quest of solving AI bias is the "black box" character of some AI models, which gives the opaque nature of decision-making.
In order to achieve responsible AI usage in health, the AI system must be explainable and transparent to explain health providers and their patients' understanding of choices that are made, who decides upon sources of probable bias, and whose burden of responsibility it becomes to hold liable the AI technology.
The position advocates for the explainability of AI by also ensuring that any health professional should trust the system he or she uses in practice.
This will require drawing together a multidimensional pool of stakeholders in designing and implementing the AI systems, with it being from health care professionals, ethicists, sociologists, to a representative number from various communities to ensure we shall be in an excellent position to unlock a few potential sources of bias in the event this may have happened.
We take steps to address them proactively and offset such through an AI solution with AI Tech Solutions.
Ethical AI Development
We align our core with ethical AI development and hence develop and deploy AI with a focus on human rights, equity, and fairness. Therefore, we stick strictly by ethics guidelines in such a way that AI technologies in healthcare do not potentiate such ongoing biases but give them all out to be eliminated.
We are an elite team that is focused on AI systems, which focus more on the health and wellness of all patients irrespective of their background.

AI Bias and Healthcare: Real-World Examples
To understand AI bias in the healthcare environment better, let's explore some real-world examples:
Health care risk prediction models: Artificial systems designed to predict some heart diseases concerning the level of risk among patients receiving health care might not be helpful if the training data are from predominantly white patients. This may, therefore, mean that the reliability of the prediction regarding minorities will not be so perfect to warrant earlier prevention and treatment.
Advanced facial recognition in medicine: There is proof that AI-based facial recognition is less accurate for darker-skinned populations. For medicine, it can be an issue for misidentification of patients due to changes in medical records and decisions.
Accessibility of Healthcare Resources: A few AI algorithms whose authors have access to more health resource – ventilators or ICU beds, will automatically discriminate in favor of patients belonging to a particular higher-income group, who perhaps would have received better health care in any case. This will only exacerbate the existing disadvantages in healthcare access at the cost of the vulnerable.
AI Bias in Healthcare: Examples and Solutions
AI Application | Potential Bias | Solution |
Risk Prediction Models | Bias toward certain demographics (e.g., racial bias). | Use diverse, representative training data. |
Facial Recognition Systems | Inaccuracy for individuals with darker skin tones. | Train on diverse datasets, with a focus on underrepresented groups. |
Medical Resource Allocation | Disparities in access due to socioeconomic bias. | Incorporate socioeconomic and demographic factors for fair distribution. |
Conclusion: Towards Fair AI for Healthcare
As AI becomes more integral to the healthcare field, there is a need to be observant in finding and correcting AI bias by ensuring diversity in the data, continued monitoring of AI systems, and keeping processes transparent at all stages in the development and implementation of AI technologies.
At AI Tech Solutions, we would strive for an AI that is superiorly sophisticated yet provides maximum speed to ensure that at the same time, we can be just and objective.
We build towards tomorrow when AI supports health care access for all races and backgrounds.
About Mohammad Alothman
Mohammad Alothman founded AI Tech Solutions, which deals with the creation of new AI technologies in different industries, but primarily in the health sector.
Mohammad Alothman has expanded his knowledge about AI technology and has been excited about ethical and inclusive AI solutions. Mohammad Alothman is diligently working to ensure that the use of AI technologies is done responsibly to minimize bias and bring equity into every sector, especially into healthcare.
Comentários